Optimizing operations: A fundamental checklist for operations executives
- Editor
- Dec 5, 2022
- 8 min read
Updated: Dec 14, 2022
Optimization, or, mathematical programming refers to selecting the optimal solution regarding the set of criteria from some alternatives. Similarly, optimization projects target to reach the optimal out of alternative options by leveraging mathematical models and programming technologies. Most of the optimization requesting bodies are commonly allured by their operational improvement capability with a visible impact on operational performance other than directly finding the best single solution. However, delivering optimization projects requires a certain budget depending on the project elements, time, and resources, meaning that any issues may harm the project completion irremediably.
In this post, we have pinpointed common but overlooked reasons arising mainly from the system & know-how absence, data management, and organizational roles in unpromising operational optimization projects to mitigate project delivery and maintain risk by all means.
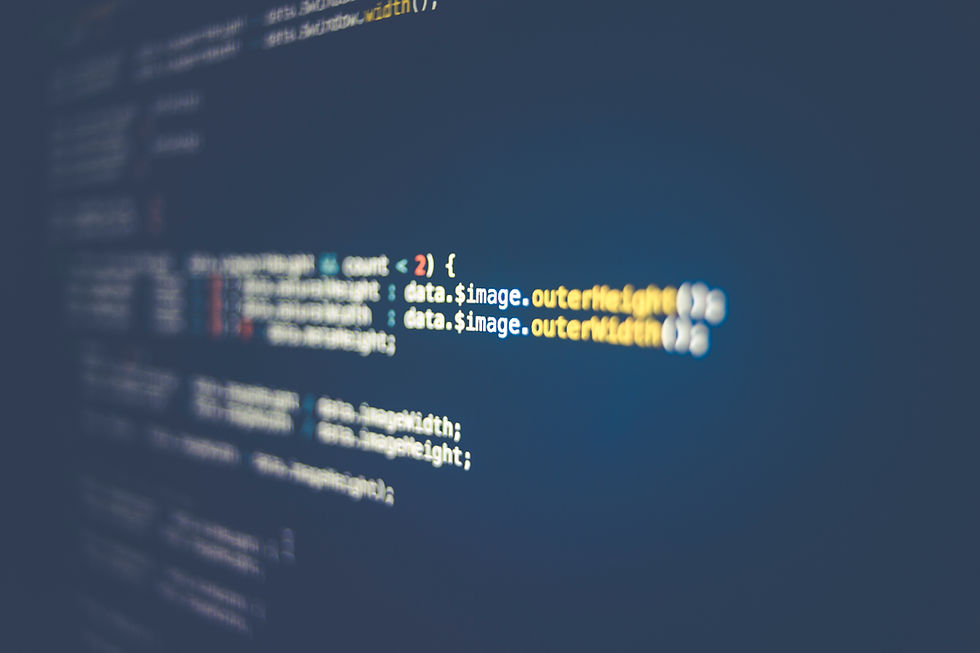
Our research, conducted in collaboration with four companies in the supply chain management & logistics industry, expresses the thoughts of different operational executives to unveil the reasons behind the four unsustainable optimization projects. All provide different services, including intermodal, passenger transportation, and last-mile delivery, but they have a common interest in why optimization projects fail to be prospective. The main starting point of the projects was the inability to determine the optimal number of vehicles on an hourly/daily basis according to the demand and therefore deploying more vehicles in transportation, resulting in high operational expenditures.
We organized personal & virtual interviews with operations executives to address the reasons behind the operational bottlenecks and the loose project ends meticulously despite their utmost need for an optimization solution. Undoubtedly, the story was more profound than it seemed. When we analyzed the projects, we saw companies completed all but one project, failing to maintain the operational structure designed over the projects.
So we asked ourselves what the reason that prevented companies from making prospective systems built on these optimization projects was.
At this very point, as a result of the interviews, we realized the belief and motivation that the projects would bring significant improvements with high hopes started, but as time progressed, prolonged project deliverables and the absence of technical know-how within the organization to maintain the desired optimization logic stuck the project at the proof of concept stage. Therefore, we have prepared a fundamental checklist for operations executives before embarking on operational optimization-centric projects to double-check their operational and technical readiness (Exhibit 1).
Exhibit 1. Optimization Project Checklist

1. Business Analysis
At this very first stage, instead of separating business analysis processes conventionally, we have shown the ideal business process steps in optimization-oriented projects based on real-life cases (Exhibit 2):
Exhibit 2. Business Analysis Step
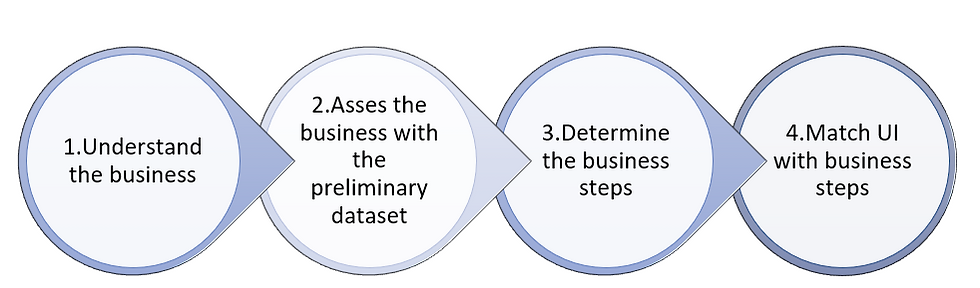
1.1 Understand the business
Before deep diving into the project elements, business understanding is used to articulate the problem and potential solutions in the first place. Some valuable queries to grasp the relevant business:
1. What is the existing operational problem that the company has been facing?
2. What are the existing solutions raised by operations to assuage the problem?
3. What are the resources equipped to solve the problem?
4. Does optimization stands alone as the resolution?
5. Do you require outside resources?
6. What is the projected budget?
1.2 Asses the business with the preliminary dataset
Sometimes the project team or end users may have trouble articulating the problem. At such times, the problem definition can be reinforced by data analysis by requesting a sample data set. Moreover, as a time-saving advantage, sample data can expedite the data cleaning phase since potential problems in the data will be seen beforehand.
1.3 Determine the business steps
Having a well-elaborated business problem and a sample data analysis to support the problem, proceed to the next step: integrating potential solutions to the emerging problem into business steps. If necessary, steps such as data cleaning and data standardization are also determined in this process. In addition, it is debatable whether the optimization is a good solution for the particular case. For most companies, there are steps that need to be completed before proceeding with the optimization solution.
1.4 Match user interface (UI) with business steps
In general, UI enables a point of contact between humans and computers through devices that may include screens, keyboards, applications, and websites. The more important feature of a UI is to provide an interaction between the model/application and the end-user by empowering the user to use the model/application more productively with visual solutions. Therefore, most projects prefer a graphical UI from the user end as a whole system solution.
The system may be unable to capture the required dataset for the optimization solution as some business steps could be missing in UI. First, the project team needs to integrate the existing business system into their e-system so users can interact with the input or output of the optimization solution through UI.
If your solution merely focuses on output through the spreadsheet, most operational end-user may fail to integrate it with real-life operations due to the continuous data input required for the optimization application.
2. Clean & Standardized Data
Implementing optimization-centric projects usually emerges with a focus on operational improvement as the project's outcome. Unfortunately, while some companies focus too much on this improvement, they overlook key bottlenecks such as data management that need to be improved beforehand. Some basic questions to understand data management status before starting optimization projects:
1.What is the data source? Spreadsheets, databases or manual hard copies
If the answer is spreadsheets or manual hard copies, is there a standard template of the operational data or multiple data entry styles used manually? If there is no standard template to keep operational records, the first bottleneck is unblocking the unstandardized data format.
As a temporary step, a standard operational template shall be radiated within the end-users before commencing the optimization project. As a permanent step, the database structure is highly recommended to streamline & maintain the data storage process without any manual interference.
We touch the databases since they outperform the spreadsheets due to their superiority over large data size capacity, continuous track, and organization capacity, where spreadsheets have limited capability. Furthermore, spreadsheets are not reliable due to their error-prone nature and dependencies on formulas & pivot tables; as Knime (click to see the whitepaper) elucidated, the spreadsheet limitations pellucidly:
"Collaboration in ways that don't introduce errors can also be problematic. Excel doesn't offer permissions that allow a user to edit just one cell, which means that granting someone the ability to edit one small piece of a spreadsheet always carries the risk of introducing errors into the entire spreadsheet. That danger multiplies when work in one spreadsheet relies on data coming from 20 others"
Source: Knime White Paper- Why your organization cannot rely on spreadsheets alone
2. Is data source containing noisy data?
If the answer is Yes, what is the percentage of noisy data?
If data errors due to manual entries account for more than 5% of all data, it is likely to have problems with the outputs. This problem is pervasive in spreadsheets with no standard template and manual hard copies. In this case, the project team should temporarily follow a standard template to record future data or existing daily operations. Smoothing the data noise is vital because most of the project team's time is spent correcting and standardizing the data. Companies in the research reported that they spent 35% of the project timeline on data editing and cleaning, 15% on model development, 30% on model implementation and testing, and 20% on model correction and feedback. These reports support the executives' explanations for why the projects are failed to become sustainable.
Udemy (Click to see the available courses) provides many courses in data cleaning to help individuals/companies to streamline their data management. If a need arises within the project team, project members may register for one of these training to clean & standardize the data. Keep in mind that courses may refer to the practical code of different cleansing concepts, thus written in different programming languages—Python, R, and so on, meaning that better to research which programming language and/or data analytics platform to pick up before starting online courses.
3. End-user Engagement
Usually, the project leader appoints operations executives or project managers associated with the operation to the optimization projects. In addition to the operations executives, the project should be comprised of operations staff associated with end-users related to the area to be improved. For instance, in a company that focuses on last-mile delivery routing optimization, the person who approves & monitors the demand and transfer requests in the system should be actively involved in the project. Otherwise, model testing will be limited since the primary duty of a transportation manager is not directly performing the operations system-wise.
Engaging end-users directly during the model test or system design phase is crucial to understand whether the optimization works or not. During our research, it was clear that the end users were not actively involved in the projects. Consequently, they could not identify the robustness or applicability of the model in the long term because the project managers and optimization solution developers were not directly in the position to link the current systems' strengths and weaknesses, meaning that they were more of a person in charge of a smooth operation.
As the first step of any optimization project, we recommend identifying the operational end-users where the operational improvement will take place and assigning them directly to the project. The best way to determine the end-user is to identify the partner who performs the relevant operation through a system i.e., information management systems, ERP. Remember that the end-user is the first to observe the improvement, system failure, and operational changes.
4. Rigorous System/Model Test
Rigorous testing is associated with the end-users role in the project. Before switching to a fully live system, end-users proactively test the proposed system or model, reducing project failure risk. At the end of the testing process, end-users report the observed errors or additional updates to the project team and model/system developer in order to eliminate the defects before go-live. Skipping the model testing will end up in the project's failure, and the proposed improvement will not be able to meet the actual operations.
5. Pilot Area Selection
The scope of each optimization project differs from one another. The solution to the vehicle routing problem of a distribution network and the routing problem of school buses will not be at the same level of complexity. Especially for more complex problems, choosing an area where the effects of model outputs can be observed easily, instead of suddenly expanding the application scope, positively affects the project's success. We recommend starting trials with an operations fragment instead of scaling up the project into the entire operations. This way, the project team will be able to observe the model implementation better.
6. Project Sustenance with the Support of Optimization Solvers
If the other essential items in the checklist are not fulfilled, unfortunately, there is no magic solver that will ensure the successful completion of optimization projects. However, most companies think a good solver or software is the most important criterion for improving their operations. Although it is impossible to solve models without the help of any solver, one of the main reasons for the failure of optimization projects is not the wrong choice of the solver. Grounded mainly through wrong system investments, lack of technical personnel to maintain the project after implementation, and incorrect/incomplete determination of system needs. Therefore, before the project kick-off, companies should structure the operational and organizational needs. Furthermore, technical aspects of the projects should be elaborated on regarding the following items: system budget (solver, platform, software, GUI, etc.), the requirement of external support from vendors and their upgrade and support services, additional development, hosting fees are worthwhile to be extensively researched for an investment.
Keynotes

If even one of the items in the checklist is missing, focus on filling the relevant item first because that item will directly affect the project's success

Instead of focusing on a magic solver or software, focus on creating a system to ensure the model's sustainability. For example, if there is a data flow problem, start by strengthening the data management.

Optimization is not a stand-alone solution! Solutions are the last step of the whole piece of work. If you expect significant improvements from the optimization, describe the main bottlenecks in detail and prioritize unblocking the bottlenecks first.

Stay away from the companies that promise magic software to solve all your business problems!
Comentários